How Spotify’s AI-Driven Recommendations Work?
Spotify, the world's largest music streaming service, is famous for personalized playlists via its recommendation algorithm, yet criticized for algorithmic bias. It uses collaborative and content - based filtering, but faces issues like bias reinforcement, cold start for new artists, and cultural bias in metrics. In future, it introduced an AI DJ and is exploring reinforcement learning to enhance user experience.
- Author: Shine
- Update: Apr 02, 2025
Spotify's AI - Powered Playlists: Innovation and Controversy
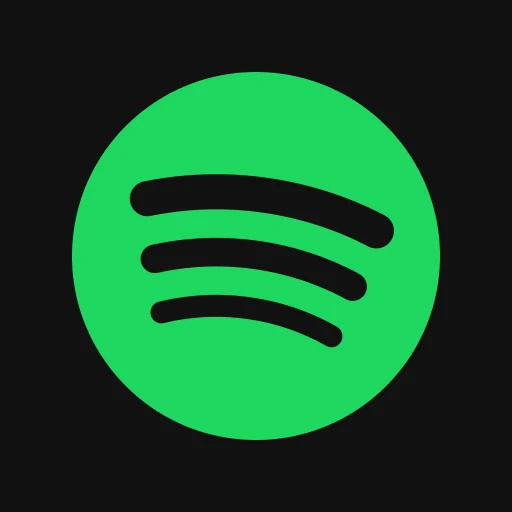
Listen to endless music, podcasts, and playlists on-the-go.
Spotify, boasting 500 million monthly users, stands as the world's largest music streaming service and the hub of audio. It's renowned for its personalized playlists crafted by its recommendation algorithm. Considering users as raw material, on top of the data layer, shared models can be constructed. However, this heavy reliance on artificial intelligence has attracted criticism from some industry experts concerned about algorithmic bias. Let's explore how Spotify employs AI to personalize user experiences on its platform and the technology underlying it.
The Evolution of Spotify’s Recommendation Algorithm
Back in the early 2000s, people often found music recommendations through top charts and early streaming platforms such as Pandora and Last.fm. When Spotify entered the scene in 2008, it wasn't the first to use analytics for music recommendations. But it combined various computational techniques in a unique way to make its recommendations seem more lifelike.
Thomas Hodgson, who studies algorithms and artificial intelligence, especially focusing on how new technology from music streaming companies affects artists, notes that fans describe playlists like Discover Weekly and Daily Mix in very human - like terms.
In 2014, Spotify acquired The Echo Nest, a music analytics firm. This acquisition blended machine learning and natural language processing to build a database of songs and artists, marking an important step in the evolution of its recommendation system.
Using User Data to Create a Map of Songs and Artists
The system starts with collaborative filtering. This process examines patterns across all user data to understand when tracks are often playlisted together. It's like building a map of music and podcasts. In this map, each point represents a different track in Spotify's catalog, and its location is determined by collaborative filtering. So, if two songs are frequently playlisted together, they'll be close in the map; if not, they'll be farther apart.
However, recommendations based solely on collaborative filtering aren't flawless. For instance, during holidays, Mariah Carey's "All I want for Christmas is You" might be playlisted more often with "Silent Night" despite their different musical styles. If Spotify only made recommendations based on proximity, users who like Mariah Carey might get recommended "Silent Night" when they aren't interested in Christmas carols.
Content - based Filtering Tech
To address such issues, Spotify adds content - based filtering. This algorithm collects metadata like release date and label and conducts a raw audio analysis. It uses metrics such as dance ability and loudness to describe a track's sonic characteristics. For example, "Uptown Funk" has a dance ability score of 0.856 on a scale of 0 to 1. The algorithm also analyzes a track's temporal structure, as seen in the visual representation of Taylor Swift's "Anti - Hero". Content - based filtering also considers a track's cultural context by studying lyrics and analyzing adjectives used to describe the track in articles and blogs.
While these filtering techniques aren't exclusive to Spotify, industry experts say the platform stands out due to the vast amount of user data it has and the products it creates from that data. Spotify claims its content - based filtering technology has evolved over time and now includes more advanced proprietary features.
The Dangers of Algorithmic Bias
Hodgson warns that algorithms could reinforce existing biases. For example, if a music catalog has more male than female artists, as listeners engage with it, these biases could be magnified, creating a feedback loop. Spotify states that its research teams evaluate and mitigate potential algorithmic inequities and harms, striving for transparency.
Another criticism is the cold start problem, where new artists lack user data, and thus the algorithm isn't optimized for them. Sultan points out that human editors play a significant role in such cases as they are some of the best at understanding new releases, culture, and relevance. However, Hodgson's bigger concern is that certain metrics in the platform's audio analysis might be culturally biased.
For example, Spotify's algorithm labels a North Indian classical track's key signature as E minor, which is inappropriate for that musical tradition. Spotify contends that audio analysis is just a small part of the overall system, which considers many factors before making a recommendation. Some industry experts also highlight issues with how the system understands metadata for classical music, such as the complex metadata for a Tchaikovsky track. Apple Music, a recent competitor to Spotify, released a new app in March to address such problems, but Spotify doesn't comment on a competitor's marketing campaigns.
Spotify’s Future Plans in AI
In February, Spotify joined the generative AI trend. Its AI DJ gives the algorithm a human voice and provides listeners with additional context around recommendations. Sultan reveals that the company is also exploring reinforcement learning. This technique would enable the recommendation system to learn automatically based on feedback, helping with recommendation diversity and long - term user retention as Spotify aims to push the boundaries with new technologies, capabilities, and experiences.